Mathematical Theory for Adversarial Deep Learning
发布时间:2022年10月28日
浏览次数:4307
发布者: Wenqiong Li
主讲人: 高小山(中国科学院数学与系统科学研究院)
活动时间: 从 2022-11-01 16:00 到 17:00
场地: 线上
Abstract: Deep neural network (DNN) is the central tool for the current revolutionary progresses of artificial intelligence. However, the existence of adversarial samples makes DNN vulnerable in safety-critical applications. Although many effective methods to defend adversaries have been proposed, adversaries are still inevitable. In this talk, I will present some progresses on the mathematical theories for adversarial deep learning, including the existence of robust DNNs for a separated data set, the information-theoretically safe bias classifier against adversarial attacks, and finally a how to achieve optimal robustness against adversarial attacks using Stackelberg games.
报告人: 高小山,中国科学院数学与系统科学研究院研究员,主要研究数学机械化与人工智能数学理论及应用。曾获国家自然科学二等奖、香港求是杰出青年学者奖、吴文俊应用数学奖、吴文俊人工智能杰出贡献奖、国际计算机学会ISSAC杰出论文奖。
Tencent Meeting:
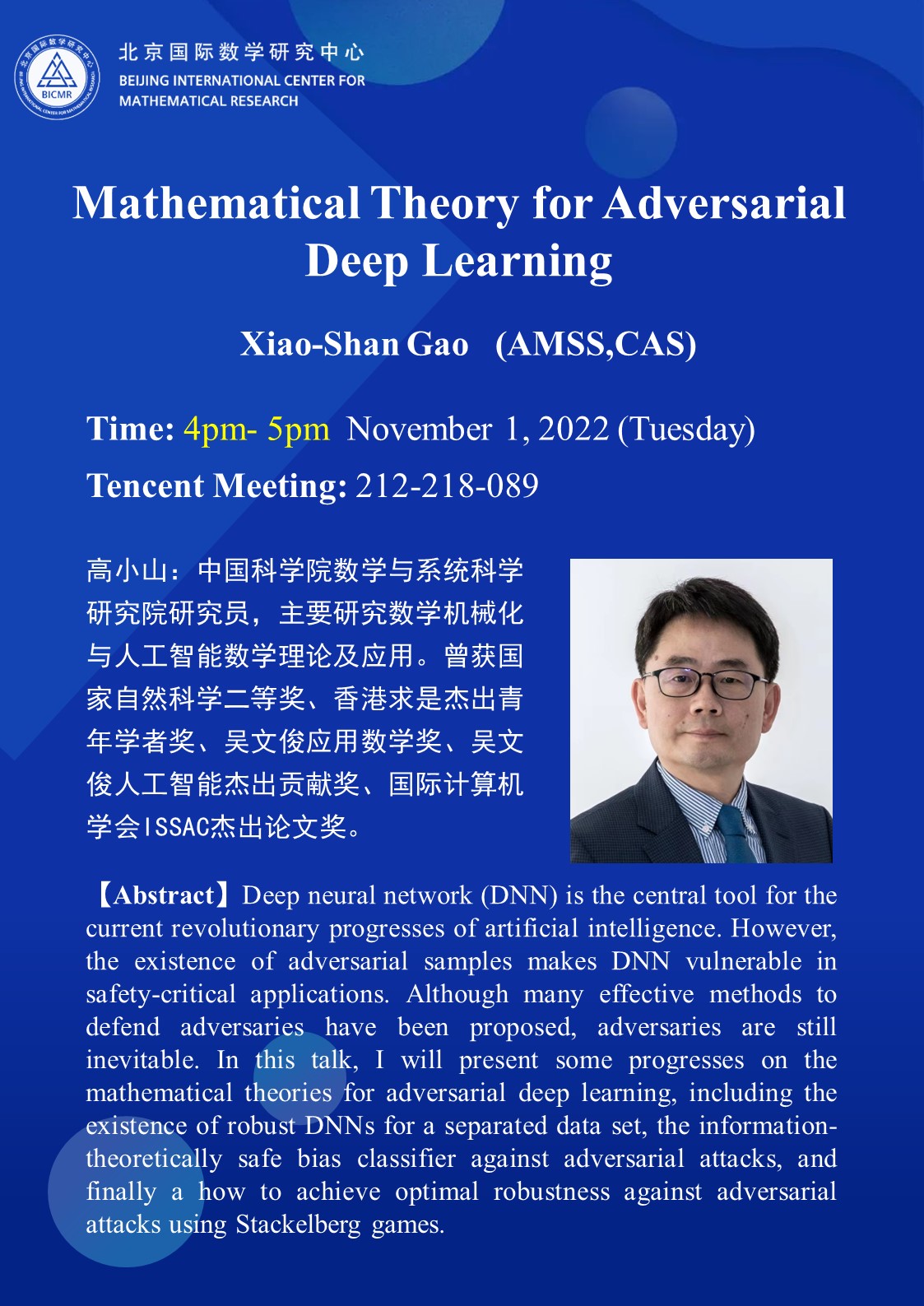